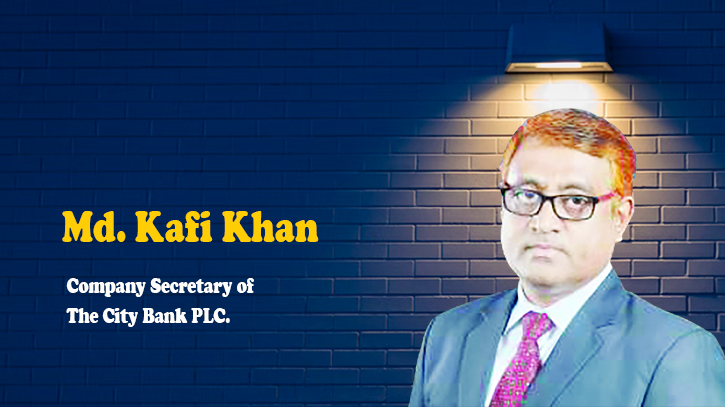
Photo : Messenger
ChatGPT is a powerful language model created by OpenAI. It uses advanced machine learning algorithms to generate human-like responses to user queries and inputs. Chat GPT is a language model capable of performing various language-related tasks. Among them are language translation, question answering, and content generation. It has been trained on a vast amount of textual data. Hence, Chat by Open AI can provide accurate and contextually relevant responses to various queries. Chat GPT is used in multiple applications, including chatbots, virtual assistants, and content creation tools.
ChatGPT can help financial institutions detect and prevent fraudulent activity and other potential risks. For example, a bank could use ChatGPT to monitor customer accounts for suspicious activity. This activity includes large transactions or transfers to high-risk countries. Where there is a hammer, every problem looks like a nail. ChatGPT may be an impressive AI hammer, but not every tool is perfectly suited for every job. ChatGPT is one of the latest technology crazes to hit almost every business. Banking is no exception. Some bank and credit union executives see this generative AI model as a panacea of sorts. True, it can give some very clever responses to customer requests, sparking many people’s imaginations regarding the number of different processes it could potentially automate. Inevitably, one is customer service. However, there are a number of different concerns that should be addressed concerning why a generative AI model like ChatGPT might not be ideal for customer service roles.
Generative AI works by identifying patterns within the massive bank of data it’s been trained on to respond to queries on-the-fly. Other common chatbot models draw from a predetermined library of responses. By contrast, every response from a generative AI solution will be unique because the system takes slightly different approaches for each input. Such capability can seem fantastic if it is looking for some help writing a poem or adjusting the tone of an email. However, it’s less impressive if need system to be able to tell someone the interest rate on a specific credit card. When it comes to customer service at banks, it’s better to make sure that users are getting a consistent response every time for the same question, no matter how it’s asked.
There are many benefits to using ChatGPT in the banking industry. These include improved customer service and support, the ability to handle complex financial goals and investment decisions, and the potential to reduce costs and increase efficiency through automation say advantages are One of the primary benefits of ChatGPT is its ability to provide personalised customer service and support. By analyzing large amounts of data, ChatGPT can quickly and accurately respond to customer inquiries, providing real-time assistance and support. This can lead to higher customer satisfaction, lower customer churn, and increased customer loyalty.
Another benefit of ChatGPT for finance is its ability to handle complex financial goals and investment decisions. By using machine learning for financial analysis and providing insights, ChatGPT can help financial advisors and investment managers make informed decisions about their clients’ portfolios, taking into account a wide range of factors such as risk tolerance, investment goals, and market trends.
Finally, ChatGPT can help financial institutions reduce costs and increase efficiency through automation. By automating routine tasks such as customer service inquiries, ChatGPT can free up human resources for more complex and strategic tasks, reducing costs and increasing productivity.
The potential of generative AI seems boundless, but the truly transformative applications in banking are still unknown but two plausible versions emerge. On the more radical end of the spectrum, we foresee a potential future scenario where banking value chains undergo a dramatic overhaul. AI-powered agents would assume a central and proactive role in decisions such as uthorisation of payments, while investigative processes would become fully automated or replaced. The more probable scenario, however, is an evolution rather than a revolution. Banking value chains would remain familiar, but banks would channel their energies into applications, crafting a multitude of innovative generative AI use cases to streamline the user experience for both consumers and employees. In this vision, generative AI would become an integral, largely unnoticed component of daily life where users, recognizing the substantial advantages the technology brings, are “trained” to tolerate its limitations.
Most banks are still in the early stages of deploying generative AI and have yet to make significant progress in its adoption. Still, we have seen leading players proactively exploring opportunities. Their immediate-term focus has been on developing lower-risk, internal-facing applications that demonstrate tangible productivity benefits in day-to-day operations. To secure a competitive edge in the evolving landscape, banks are at a crucial juncture in determining where and how to begin. Here are four actionable steps for banks today: With the surge in interest in generative AI, banks often find themselves inundated with potential applications.
Traditional prioritisation methods, conducted without a practical grasp of the technology, can lead to misguided decisions. To identify truly impactful opportunities, banks must build a clear understanding of the capabilities and limitations of generative AI. They must also adopt a practical, hands-on approach to rapidly test feasibility through actual use case experiments, enabling them to assess risk and validate business cases. This approach ensures that investments are placed strategically.
Banks must establish a robust risk and control framework, setting guardrails firmly grounded in a comprehensive understanding of AI risks and their potential consequences. However, existing risk management processes need an overhaul to effectively manage the risks emerging from generative AI. These processes often focus on traditional model risk metrics such as minimising error rates, an approach that may be myopic. Organisations must recognise the long-term impact of generative AI on their standing and prioritise the management of reputational and legal risks. To expedite their journey into generative AI, banks must adopt a more balanced, multi-speed approach, facilitating safe and fast experimentation for key teams tasked with building prioritised use cases in the immediate term.
Simultaneously, they must ensure that their technology infrastructure and proprietary data are ready for the longer term to power future modelling requirements with the required flexibility, while also prioritising aspects of security and privacy. The demand for generative AI skills is expected to outpace the available talent pool. To bridge this skills gap, organisations must proactively institute upskilling and reskilling initiatives.
Generative AI has surged in the past year, with tech giants driving innovation and regulators emphasising safe, ethical, and secure development. The future for banks in generative AI promises transformative potential, necessitating immediate action. Organisations must identify impactful use cases, balance risk and governance, accelerate technology readiness, and rapidly upskill talent. True leadership requires foresight, trend discernment, and the agility to adapt innovations locally. As generative AI cements its place, organisations need to act swiftly to position themselves at the forefront of this revelution.
The writer is the Company Secretary of City Bank PLC.
Messenger/Fameema